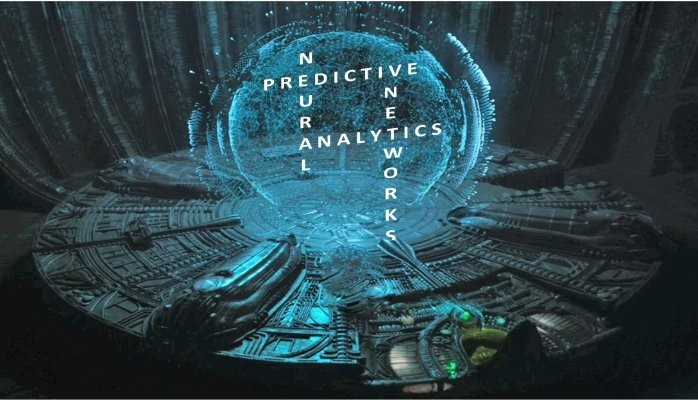
Spread the love
From the past few years, the need for automation is increasing. This may be due to a growing demand of building reliable and efficient systems to scrutinize an enormous amount of data. The field of machine learning utterly caters to such demands. The history of machine learning can be traced back to the mid-twentieth century wherein it was developed as an integral part of Artificial Intelligence. It basically relies largely on computational statistical theory.
Machine learning can be perceived as an algorithmic approach which imparts human intellect to machines by way of different methods for learning, training, testing and prediction purposes. It plays a pivotal role in Human-Machine Interaction (HMI) and finds application in a wide range of industries – healthcare and medicine, affective computing, call centres, education, automobile, entertainment. Hollywood movies such as “The Bicentennial Man”, “I, Robot”, “Real Steel”, “Transcendence” have used the similar concept.
A question arises that how machine learning takes place in practice? In general, when we think of machine learning, the first thing that strikes our mind is a machine behaving just like a human being. One such paradigm of machine learning that aims to mimic the functioning of human brain is the Artificial Neural Network (ANN). The human brain can be considered as the most advanced and extensively developed organ of human anatomy. So, how does a machine acquire knowledge or how does it “learn” like we humans do? The answer to this is that we learn most of the things through our past experiences. In a similar way, ANN grasps knowledge through a large amount of pre-recorded data.
Identical to a human brain, an ANN consists of massively interconnected structural processing units, aptly called as “neurons”. It has a layered structure, the input layer, output layer and a number of layers in between called as hidden layers. The ability of parallel computations, power to model non-linearity efficiently and simplicity, makes ANN a feasible choice for a majority of applications. Hence, ANN essays an important role in pattern recognition and prediction queries.
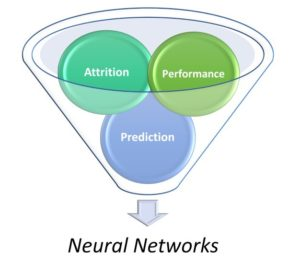
Can an ANN predict the occurrence of future events? A lot of work is going on, on predictive analytics in recent years. Organisations are focusing more on predictive analytics with a purpose to improve business quality and revenue by analysing the available resources. Predictive analytics involves anticipating the future events by closely evaluating the past data. A simple illustration for predictive analytics would be daily weather forecasting which relies highly upon predictive models created from pre-reported climate statistics. The development of such a predictive algorithm demands huge amount of data. In addition, the prediction accuracy depends upon the quality of data. The predictive algorithm should be capable of making predictions in noisy environments. All such needs can be handled efficiently by ANN. Besides, ANN requires large amount of data for proper training. Thus, ANN fits precisely for deducing a predictive model.
Predictive analytics intends to find correlation between these traits and create the desired hypothesis.From an organizational point of view, predictive analytics may be used to predict the commitment of an employee towards the company, that is, probability whether an employee will stay in the organization for long term. In the banking sector, it may be helpful to determine if the customer will be a good or a bad one depending on past records such as financial status, timely reimbursement of loan or maintaining minimum account balance. This can be achieved by analyzing the past records of the customer based on performance, work culture, rapport with team mates, etc. Currently, PMaps carries out such assessment tests with a goal to improve hiring efficiency for different organizations. In the near future, PMaps envisions to implement predictive analytics using neural networks in order to provide a cost effective and productive solution for better hiring.
– Apoorva (Team – PMaps)
